When done right, user research gives you a direct line to your customers’ real-world needs. But in an AI world, many teams are skipping this step—or doing it wrong.
This matters more than ever. With AI changing how users interact with software, our assumptions about what’s intuitive, helpful, or even ethical are up for re-evaluation.
The problem? Most users have little experience with AI tools—and most product teams don’t fully understand what good looks like yet. This gap is where the most critical research needs to happen. At the same time, designers aren’t entirely sure of best practices either. Since these tools are relatively new, product teams are still figuring out things like UI, functionality, information density, limitations, and more.
“You’ve got to start with the customer experience and work backwards to the technology. You can’t start with the technology and try to figure out where you’re going to try to sell it.”
– Steve Jobs
This quote perfectly sums up how many companies currently view AI. Rather than taking the time to understand users’ needs and build truly valuable features, they often assume what users might want, look at what competitors are doing, and go from there.
As product designers, we must approach this territory with humility. As mentioned earlier, we don’t yet fully understand what AI can truly do for us or our users—but we can begin by asking what users hope to achieve if given a way to do things more effortlessly. And then, build from there.
Below I’ve compiled 5 errors companies are making when tackling new products and features that will use AI:
1. Lack of User-Centric Design
Too often, companies focus on the technology itself (as Jobs pointed out) rather than truly understanding their users’ needs and pain points. This leads to AI roadmaps and features that sound impressive but offer little real value. Skipping user research—such as surveys, interviews, or usability testing—results in a disconnect between what users want and what you’re building.
2. Overcomplicated Products
Adding too many AI features can actually make products harder to use. Users may feel overwhelmed by functions they don’t understand or need. How many reports have you seen about Apple Intelligence or Google Gemini and their multi-million-dollar efforts to bring “groundbreaking AI features,” only for users to turn them off because “nobody asked for this”?
Avoid creating a fragmented experience where AI features live in isolation, disconnected from the rest of the user journey. Instead, integrate them seamlessly and intentionally.
3. Lack of Transparency
Users might not fully grasp how AI works—but they do know it involves their data. This leads to mistrust. Companies should aim to be transparent about how AI makes decisions and the data it uses. Keep in mind: AI models can be biased, and that bias can affect both your users and your brand’s reputation.
4. Inadequate Testing and Iteration
Yes, AI is being hyped as the next digital gold rush (just like AR/VR or NFTs were a few years ago, remember?), but rushing to release just to keep up can backfire. Poorly-tested features will frustrate users and generate negative word of mouth from these early adopters. Take the time to properly test, identify bugs, address inaccuracies, and improve performance.
If you’ve already launched AI features, use in-app surveys or email campaigns to gather authentic feedback. Let real user insight shape the future of your product.
5. Misalignment with Business Goals
Some companies add AI features without a clear understanding of how they align with business objectives or user value. Prioritizing flashy, quick wins over long-term strategy leads to unsustainable product development, rework for design and dev teams, delivery delays, and exhausted people. Ensure each feature serves a purpose—both for the user and for the business.
–
Here’s the takeaway: AI is only as useful as it is usable. If your users can’t clearly understand it, trust it, or benefit from it—then it’s just noise.
To build AI features people actually want to use, start by doing the hard but worthwhile work of understanding their real-world tasks. Let user research guide what you build, how you build it, and what you say no to.
When you ground innovation in real needs, not assumptions or hype, you don’t just build better products. You build trust.
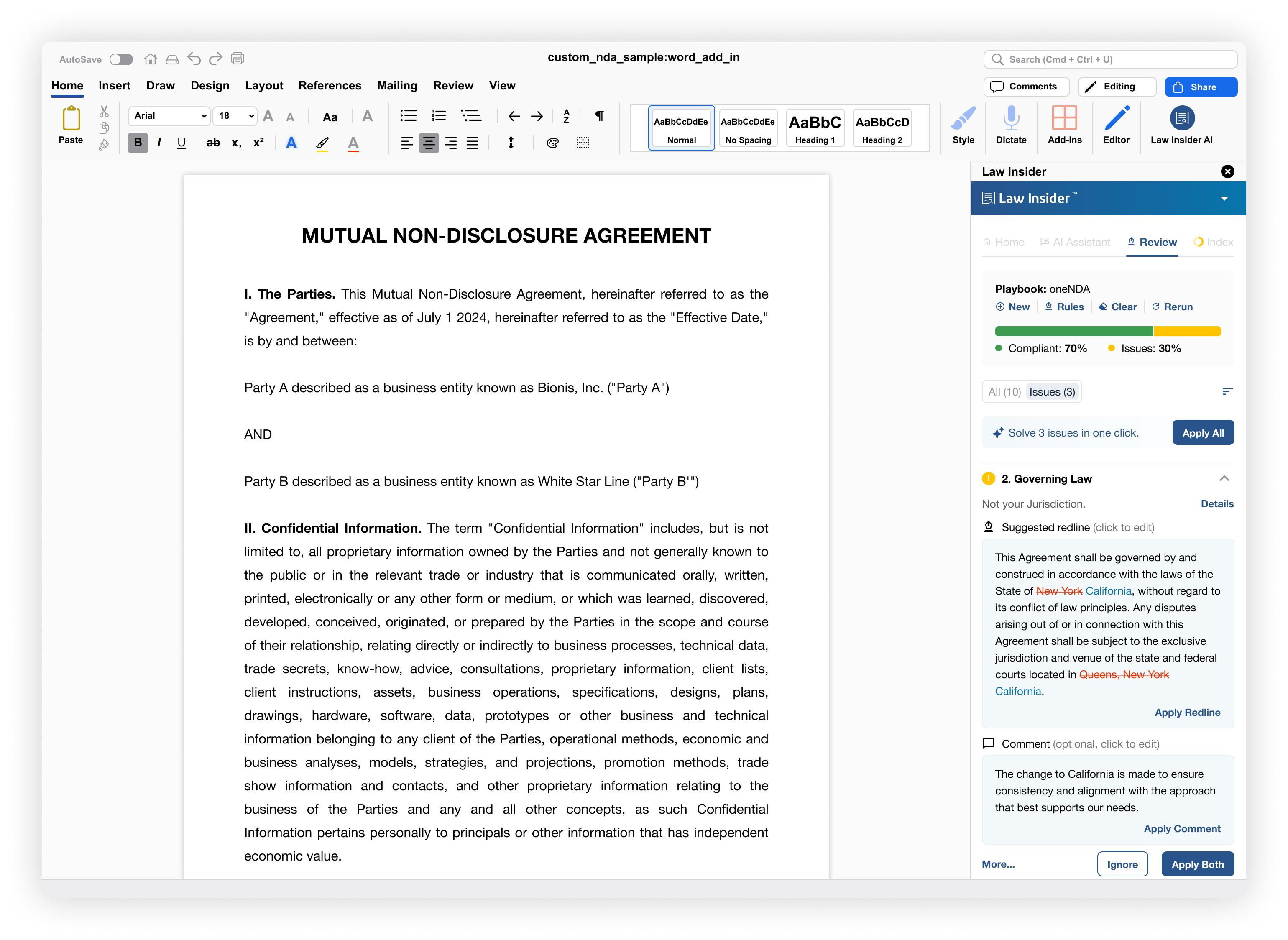